In a groundbreaking achievement, a non-invasive AI system, known as DeWave, has been developed to transform silent thoughts into text without the need for implants, requiring users only to wear a well-fitted cap.
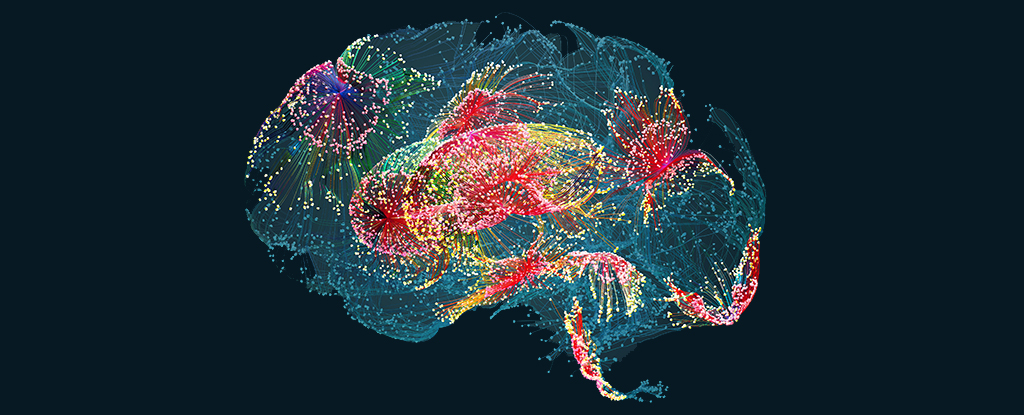
The Australian researchers behind DeWave conducted tests involving more than two dozen subjects, who silently read while wearing a cap that recorded their brain waves through electroencephalogram (EEG) technology, translating the waves into text.
While DeWave achieved slightly over 40 per cent accuracy in experiments conducted by the researchers, marking a 3 per cent improvement over the previous standard for thought translation from EEG recordings, the ultimate goal is to enhance accuracy to approximately 90 per cent, aligning with conventional language translation methods or speech recognition software.
The innovative technology holds promise for aiding communication in stroke and paralysis patients and facilitating easier interaction between individuals and machines such as bionic arms or robots.
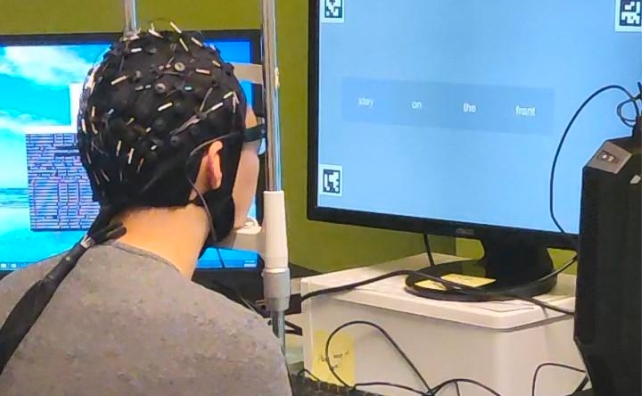
Unlike other methods that necessitate invasive surgeries for electrode implants or the use of bulky MRI machines, DeWave offers a non-intrusive solution for daily use. Traditional methods often rely on eye-tracking to convert brain signals into word-level chunks, assuming breaks between words during eye movement. However, DeWave decodes raw EEG waves into words without eye-tracking, a more challenging process.
DeWave’s encoder translates EEG waves into a code after extensive training, which can then be matched to specific words based on their proximity to entries in DeWave’s ‘codebook’. This approach introduces discrete encoding techniques in the brain-to-text translation process, presenting an innovative neural decoding method.
We think this is because when the brain processes these words, semantically similar words might produce similar brain wave patterns. Despite the challenges, our model yields meaningful results, aligning keywords and forming similar sentence structures.
first author Yiqun Duan, a computer scientist from UTS
The system’s training utilized language models incorporating BERT with GPT, and it was tested on datasets featuring eye tracking and brain activity recordings while individuals read text. This allowed the system to learn how to match brain wave patterns with words, achieving meaningful results despite challenges. Translating verbs proved to be DeWave’s strength, while translating nouns often resulted in pairs of words with similar meanings rather than exact translations. The researchers acknowledge the significance of continued efforts in the challenging endeavour of directly translating thoughts from the brain.
The research, presented at the NeurIPS 2023 conference, emphasizes the need for increased attention to encoding methods that bridge brain activity with natural language, especially in light of the rapid advancement of Large Language Models. A preprint of the research is available on ArXiv.