The most striking evidence that artificial intelligence can provide profound scientific breakthroughs came with the unveiling of a program called AlphaFold by Google DeepMind. In 2016 researchers at the company had scored a big success with AlphaGo, an AI system which, having essentially taught itself the rules of Go, went on to beat the most highly rated human players of the game, sometimes by using tactics no one had ever foreseen. This emboldened the company to build a system that would work out a far more complex set of rules: those through which the sequence of amino acids which defines a particular protein leads to the shape that sequence folds into when that protein is made. AlphaFold found those rules and applied them with astonishing success.
The achievement was both remarkable and useful. Remarkable because a lot of clever humans had been trying hard to create computer models of the processes which fold chains of amino acids into proteins for decades. AlphaFold bested their best efforts almost as thoroughly as the system that inspired it trounces human Go players. Useful because the shape of a protein is of immense practical importance: it determines what the protein does and what other molecules can do to it. All the basic processes of life depend on what specific proteins do. Finding molecules that do desirable things to proteins (sometimes blocking their action, sometimes encouraging it) is the aim of the vast majority of the world’s drug development programmes.
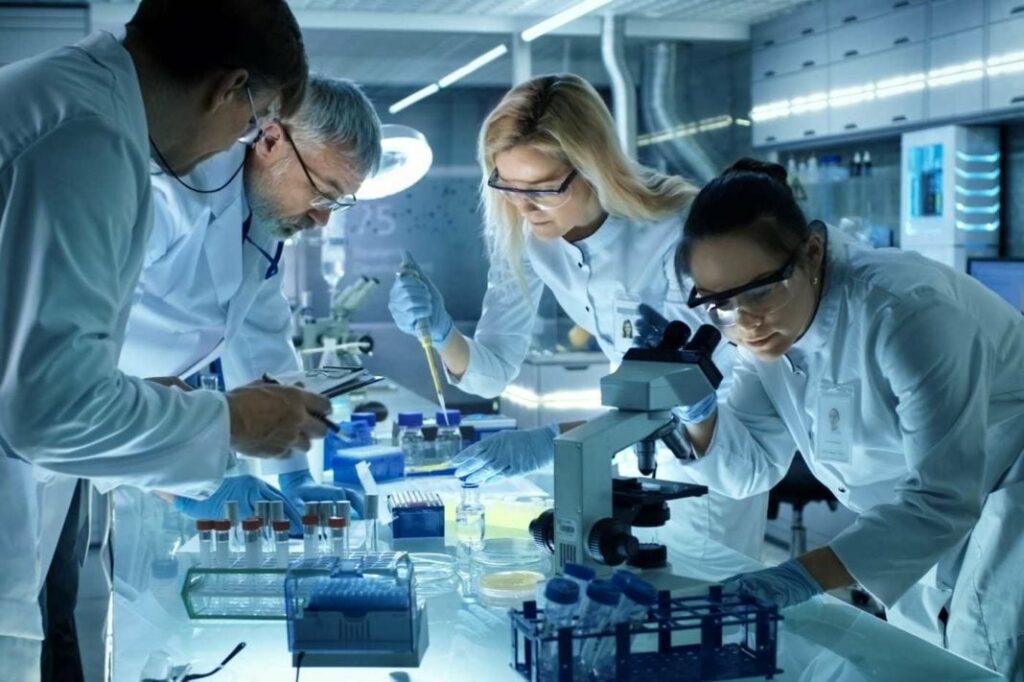
Because of the importance of proteins’ three-dimensional structure, there is an entire sub-discipline largely devoted to it: structural biology. It makes use of all sorts of technology to look at proteins through nuclear-magnetic-resonance techniques or by getting them to crystallise (which can be very hard) and blasting them with X-rays. Before AlphaFold over half a century of structural biology had produced a couple of hundred thousand reliable protein structures through these means. AlphaFold and its rivals (most notably a program made by Meta) have now provided detailed predictions of the shapes of more than 600m.
As a way of leaving scientists gobsmacked it is a hard act to follow. But if AlphaFold’s products have wowed the world, the basics of how it made them are fairly typical of the sort of things deep learning and generative AI can offer biology. Trained on two different types of data (amino-acid sequences and three-dimensional descriptions of the shapes they fold into) AlphaFold found patterns that allowed it to use the first sort of data to predict the second. The predictions are not all perfect. Chris Gibson, the boss of Recursion Pharmaceuticals, an AI-intensive drug-discovery startup based in Utah, says that his company treats AlphaFold’s outputs as hypotheses to be tested and validated experimentally. Not all of them pan out. But Dr Gibson also says the model is quickly getting better.
Crystal dreams
This is what a whole range of AIs are now doing in the world of biomedicine and, specifically, drug research: making suggestions about the way the world is that scientists could or would not come up with on their own. Trained to find patterns that extend across large bodies of disparate data, AI systems can discover relationships within those data that have implications for human biology and disease. Presented with new data they can use those patterns of implication to produce new hypotheses which can then be tested.
The ability of AI to generate new ideas provides users with insights that can help to identify drug targets and to predict the behaviour of novel compounds, sometimes never previously imagined, that might act as drugs. It is also being used to find new applications for old drugs, to predict the side effects of new drugs, and to find ways of telling those patients whom a drug might help from those it might harm.
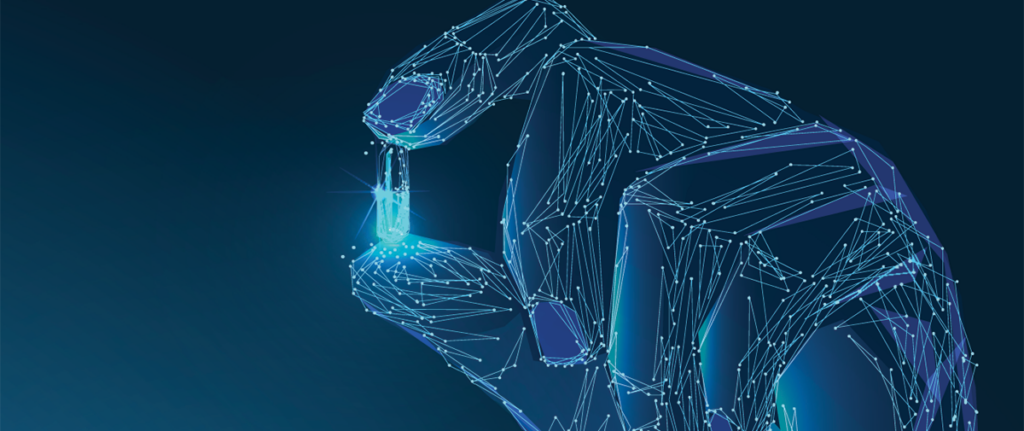
Such computational ambitions are not new. Large-scale computing, machine learning and drug design were already coming together in the 2000s, says Vijay Pande, who was a researcher at Stanford University at the time. This was in part a response to biology’s fire hose of new findings: there are now more than a million biomedical research papers published every year.
One of the early ways in which AI was seen to help with this was through “knowledge graphs”, which allowed all that information to be read by machines and mined for insights about, say, which proteins in the blood might be used as biomarkers revealing the presence or severity of a disease. In 2020 BenevolentAI, based in London, used this method to see the potential which baricitinib, sold by Eli Lilly as a treatment for rheumatoid arthritis, had for treating covid-19.
This January, research published in Science described how AI algorithms of a different sort had accelerated efforts to find biomarkers of long-term COVID-19 in the blood. Statistical approaches to the discovery of such biomarkers can be challenging given the complexity of the data. AIs offer a way of cutting through this noise and advancing the discovery process in diseases both new, like long COVID, and hard to diagnose, like the early stages of Alzheimer’s disease.