Google researchers have developed a groundbreaking weather prediction model, NeuralGCM, which synergizes machine learning with conventional methods, potentially offering accurate forecasts at a significantly reduced cost. The model, detailed in a recent Nature publication, aims to reconcile the growing divide among weather prediction experts.
Recent advancements in machine learning have shown promise in weather forecasting by leveraging extensive historical data, providing rapid and efficient predictions. However, these models often falter with long-term forecasts. In contrast, traditional general circulation models (GCMs), which have been the mainstay for the past 50 years, utilize intricate equations to simulate atmospheric changes, yielding precise projections but at a high computational cost. NeuralGCM seeks to blend these two approaches, harnessing the strengths of both.
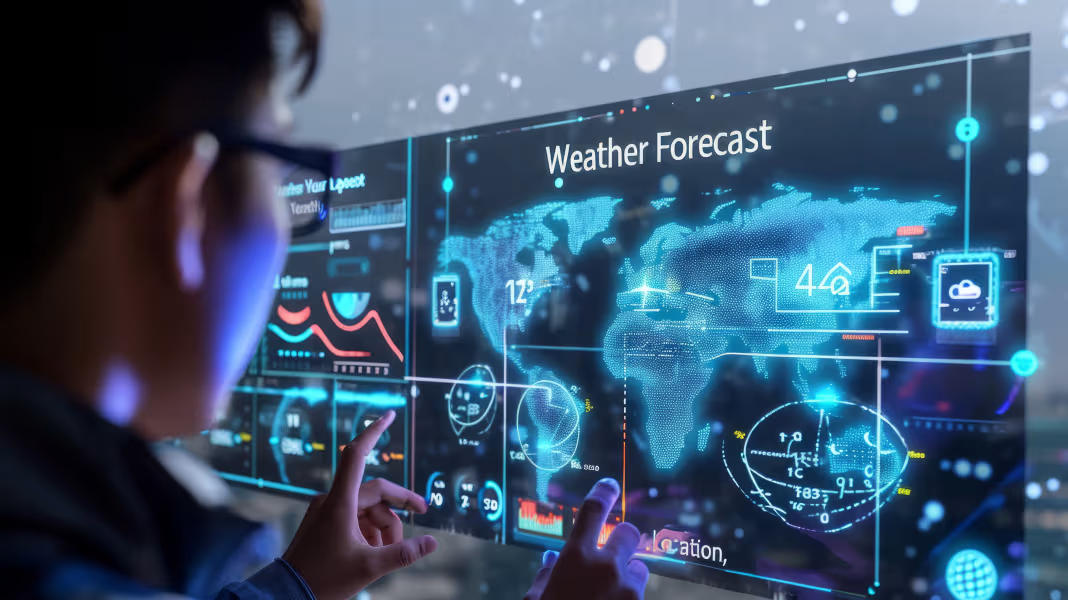
“It’s not physics versus AI; it’s really physics and AI together,” explains Stephan Hoyer, an AI researcher at Google Research and coauthor of the paper. The model uses a conventional framework to predict large atmospheric shifts and integrates AI to enhance accuracy in smaller-scale predictions, such as cloud formations or regional microclimates, where traditional models typically struggle. “We inject AI very selectively to correct the errors that accumulate on small scales,” Hoyer elaborates.
The hybrid model has demonstrated its capability to produce high-quality forecasts faster and with less computational power. NeuralGCM’s performance rivals the one-to-15-day forecasts of the European Centre for Medium-Range Weather Forecasts (ECMWF), a partner in the research.
Aaron Hill, an assistant professor at the School of Meteorology at the University of Oklahoma, sees the broader potential of such technology beyond local weather forecasts. The real value lies in modeling large-scale climate events, which are currently too costly to simulate with conventional methods. “It’s so computationally intensive to simulate the globe repeatedly or for extended periods,” Hill notes, highlighting the bottleneck that high computing costs present in climate research.
AI-based models like NeuralGCM are more compact and efficient. After training on 40 years of historical weather data from ECMWF, a machine-learning model like Google’s GraphCast operates on less than 5,500 lines of code, compared to nearly 377,000 lines for the National Oceanic and Atmospheric Administration’s model.
Hill commends NeuralGCM for demonstrating how AI can enhance specific aspects of weather modeling, speeding up processes while retaining the strengths of traditional systems. “We don’t have to discard all the knowledge we’ve gained over the last century about atmospheric behavior,” he says. “We can integrate that with the power of AI and machine learning.”
Hoyer emphasizes that while validating short-term weather predictions has been beneficial, the ultimate goal is to apply the model to longer-term forecasting, particularly for extreme weather events. NeuralGCM will be open source, inviting climate scientists and other stakeholders to utilize it in their research. This model also holds potential interest for sectors beyond academia, such as commodities trading, agricultural planning, and insurance, where high-resolution forecasts are highly valued.
Despite the excitement, Hill points out the rapid pace of AI developments in weather forecasting. “It’s gangbusters,” he says, noting the challenge for researchers to keep up with frequent model releases from companies like Google, Nvidia, and Huawei. This rapid progression makes it difficult to evaluate the utility of new tools and secure research funding accordingly. “The appetite is there [for AI],” Hill adds. “But many of us are still waiting to see what happens.”
As the research community navigates these advancements, the integration of AI and traditional methods in models like NeuralGCM could herald a new era in weather and climate forecasting, balancing innovation with established knowledge.