When a colleague visited Sam Altman in 2022 at his San Francisco home, he observed two pairs of pink high-tops on a bookshelf. One was branded with the logo of Mr. Altman’s machine-learning startup, OpenAI, while the other displayed a symbol for Helion, a nuclear fusion company he supports. More than a quirky decor choice, these shoes represent his dual passions: Mr. Altman believes that both intelligence and energy costs will decrease, supporting each other along a “long and beautiful exponential curve.”
However, the reality appears less optimistic. Utilities and data-centre operators, while excited about artificial intelligence (AI), are confronting a significant energy dilemma crucial to three major economic transitions: the AI revolution, the push to electrify various sectors, and the battle against climate change. Generative AI, like the technology behind OpenAI’s ChatGPT, consumes substantial amounts of electricity and has abruptly added to the burdens of an already strained global energy system. It’s uncertain whether there will be sufficient clean energy to satisfy all needs.
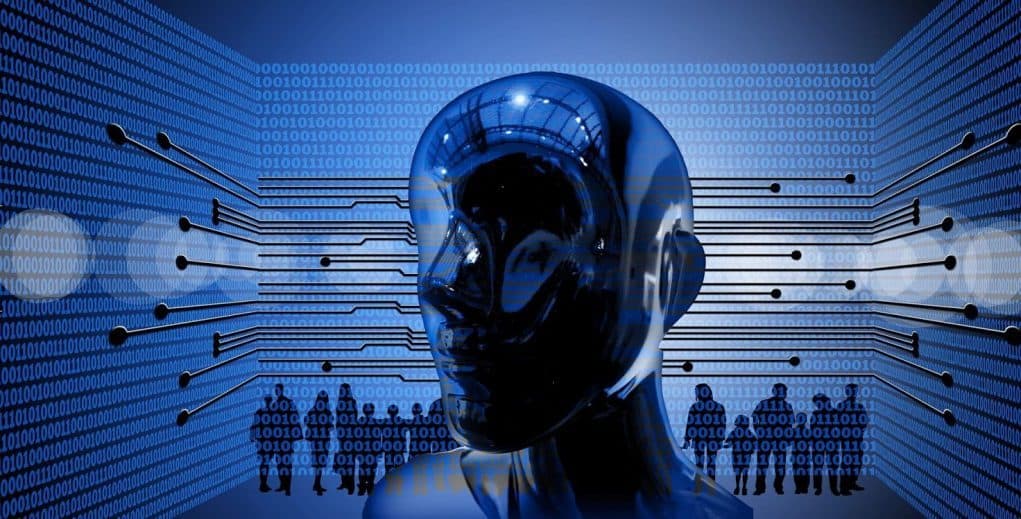
On the surface, the situation seems manageable. Data centers, utilized by companies such as Alphabet, Amazon, and Microsoft for cloud computing, have historically only used 1-2% of global energy. These tech giants have achieved significant energy efficiencies and invested heavily in renewable energy. In the U.S., electricity providers are eager to support these “hyperscalers,” having faced weak demand for electricity over the past two decades. Their executives have recently announced plans to invest billions in the next five years to increase power supply to data centres. For instance, last month, Talen Energy sold a nuclear-powered data centre to Amazon for $650m, indicating a promising start.
Yet, generative AI introduces new challenges. GPUs, essential for running models like ChatGPT, are notorious for their high energy consumption. According to Christopher Wellise of Equinix, which leases data centres, a typical pre-AI hyper scale server rack requires 10-15 kilowatts (kw) of power, whereas an AI-focused rack demands 40-60kw. Not only do the computations consume significant electricity, but cooling these GPU racks is also power-intensive. The surge in energy demand has been particularly notable over the past year from those training foundational models like GPT-4. Their broad application—from research to creative endeavours—could further stress the electrical grid. For example, a search by ChatGPT might use ten times the electricity of a standard Google search.
Predicting the exact impact of the generative AI boom is premature, but early estimates suggest a dramatic increase in energy usage. The International Energy Agency forecasts that by 2026, data centres might consume double the energy they did two years prior—equivalent to Japan’s current energy consumption. They could account for a third of America’s new electricity demand in the next couple of years. Rene Haas, CEO of Arm, suggested to the Wall Street Journal that by decade’s end, AI data centres might use up to a quarter of all U.S. electricity, a significant jump from today’s 4% or less.
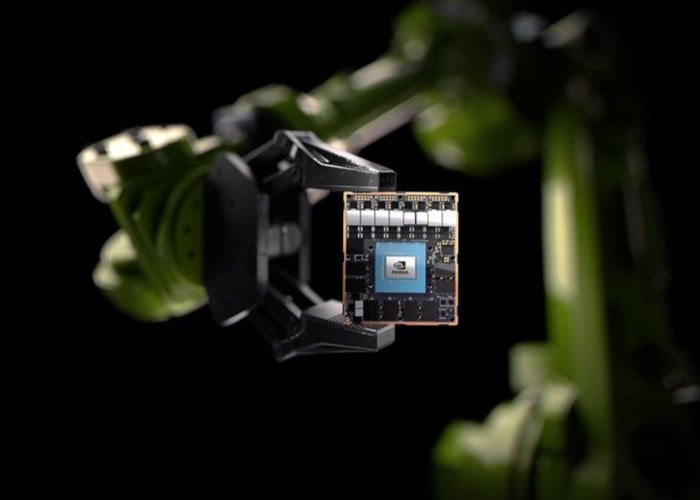
In the U.S., the challenges are compounded by timing and grid expansion difficulties. The rise of generative AI coincides with economic growth and high power demands. Zero-carbon energy is in high demand, not just from power consumers but also from sectors like electric vehicles (EVs), which continue to grow. Expanding renewable capacity quickly is a hurdle for utilities due to supply chain issues and higher interest rates, which inflate the costs of wind and solar projects. Additionally, building new transmission lines is notoriously difficult.
Creative solutions are necessary. Improving GPU energy efficiency is one approach—Nvidia claims success in this area with its latest AI servers. However, more efficient technology could lead to increased usage. Aaron Denman of Bain suggests that hyper scalers might use their financial resources to help utilities address grid limitations, particularly during peak times like hot summer days, which may necessitate standby power plants likely powered by natural gas, potentially conflicting with climate goals.
In case renewable energy falls short, the implications could be significant. The profitability of generative AI remains uncertain, and if both GPU acquisition and operational energy costs rise, it could hinder growth. Moreover, the broader electrification of the economy depends on cost-effective solutions; direct competition between AI and electric vehicles for clean energy could drive up prices and disadvantage both sectors. While optimism for Mr. Altman’s vision of fusion energy remains, it’s wise to be cautious about its feasibility.