The growing demand for machine learning and AI technologies is significantly increasing energy consumption, raising concerns about the environmental impact of these advancements. AI applications, from generating email summaries to creating unique video content, are becoming a substantial part of our digital lives, yet the exact energy cost of powering these innovations remains unclear.
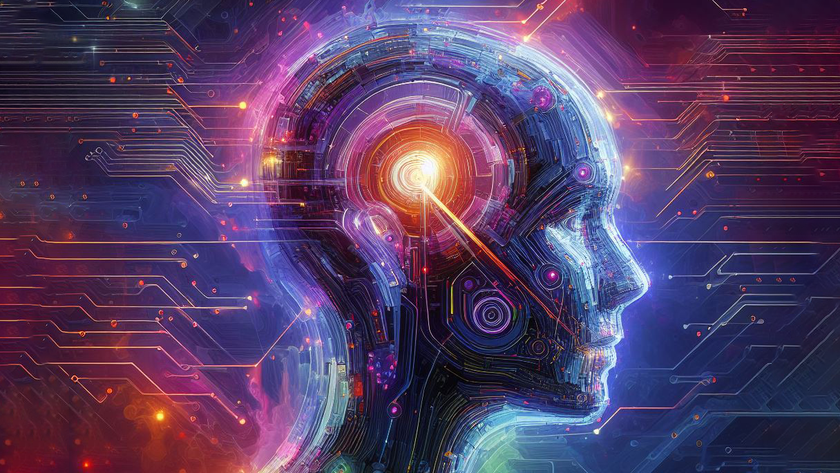
Although there are estimates of AI’s energy usage, they provide only a partial view of the total consumption due to the variability in machine learning models and configurations that can affect power use. Companies at the forefront of AI development, such as Meta, Microsoft, and OpenAI, have not disclosed detailed energy consumption data. Microsoft has indicated efforts towards quantifying and reducing the carbon footprint of AI technologies, but comprehensive data remains scarce.
A significant distinction in energy consumption exists between the initial training phase of an AI model and its deployment for user interaction. Training large models is particularly energy-intensive. For instance, the energy required to train a model like GPT-3 is equivalent to the annual electricity usage of 130 American households. This contrasts sharply with more mundane digital activities, such as streaming an hour of video content.
However, accurately gauging the energy requirements of contemporary AI systems is challenging. The trend towards larger AI models suggests increasing energy demands, though efforts to improve energy efficiency could mitigate this growth. The lack of transparency from leading AI companies complicates efforts to understand and manage the environmental impact of these technologies.
The secrecy surrounding the details of AI operations and their energy consumption is partly attributed to competitive dynamics within the industry and a desire to avoid criticism over environmental sustainability. This reticence hinders the ability to assess the true energy cost of AI applications, from the trivial to the transformative.
Research efforts have begun to shed light on the energy usage of AI models during inference, the process of applying trained models to new data. Preliminary findings suggest that while some AI tasks consume minimal energy, others, particularly those involving image generation, require significantly more power. These findings, though not exhaustive, offer a starting point for understanding the energy dynamics of AI applications.
The broader concern is the environmental footprint of the AI industry, which remains largely unquantified. Estimates suggest that AI’s energy consumption could rival that of entire countries shortly, emphasizing the need for a comprehensive understanding of and strategies to address this issue.
Proposals for mitigating AI’s environmental impact include developing energy efficiency ratings for AI models and reevaluating the necessity of AI for certain applications. These approaches highlight the importance of balancing technological innovation with environmental sustainability, urging a cautious and informed approach to the development and deployment of AI technologies.